Innovations at the Intersection: Data Engineering Meets Generative AI
In the ever-evolving landscape of technology, the intersection of data engineering and generative artificial intelligence (AI) is reshaping the way organizations collect, process, and leverage data. This convergence has unlocked new dimensions of possibilities, from enhanced data analysis to creative content generation. In this blog, we will delve into the innovative world where data engineering meets generative AI, exploring the significance of this collaboration and the transformative potential it holds.
The Role of Data Engineering
Data engineering is the backbone of modern data-driven organizations. It involves the design and construction of systems and pipelines to collect, store, and prepare data for analysis. These engineers create robust architectures that ensure data quality, reliability, and accessibility. Data engineering is responsible for transforming raw, often disparate data into a unified, structured format suitable for advanced analytics, reporting, and machine learning.
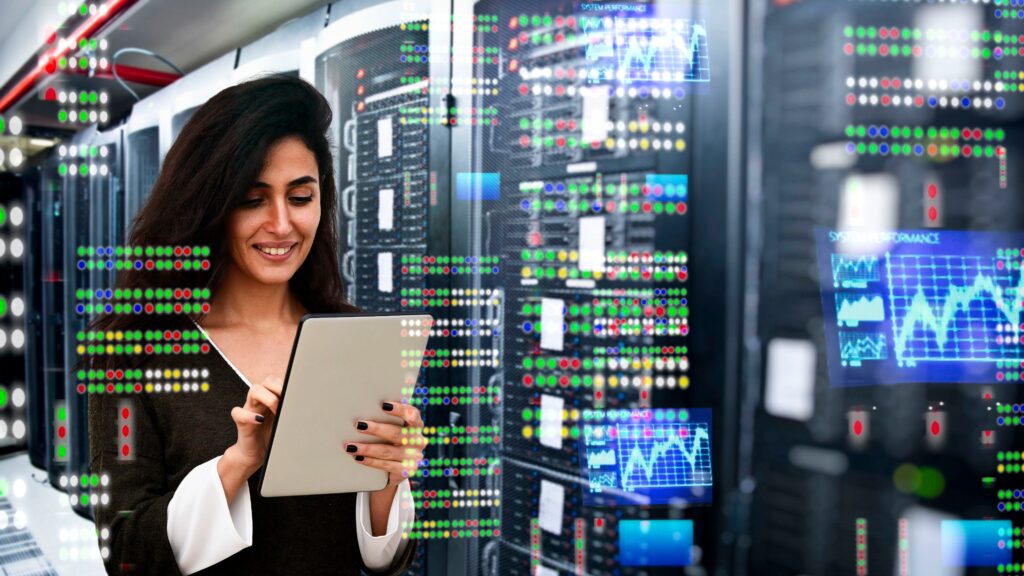
The Power of Generative AI
Generative AI, on the other hand, is a subset of artificial intelligence that focuses on creating data rather than analyzing it. This technology leverages complex algorithms, particularly deep learning neural networks, to generate new content. This content can be anything from text, images, and music to more advanced applications like architectural designs and medical research data.
Generative AI has witnessed significant advancements, notably in natural language processing (NLP) models like GPT-3 (Generative Pre-trained Transformer 3) developed by OpenAI. These models have demonstrated human-like text generation capabilities, opening the door to a wide array of applications, from content generation to chatbots and language translation.
Synergy of Data Engineering and Generative AI
The synergy between data engineering and generative AI is driving innovation in several domains:
Data Preparation and Enhancement
Data engineering traditionally involves tasks like data cleaning and normalization. Generative AI can assist in generating synthetic data to augment real datasets, thereby increasing data volumes and diversity. This can be particularly useful in scenarios where collecting more real data is time-consuming or expensive.
Content Generation
One of the most compelling applications of generative AI is content creation. Marketers, writers, and designers can leverage AI-powered tools to generate text, images, and design concepts quickly.
This streamlines content creation processes, reduces the time required, and enhances productivity.
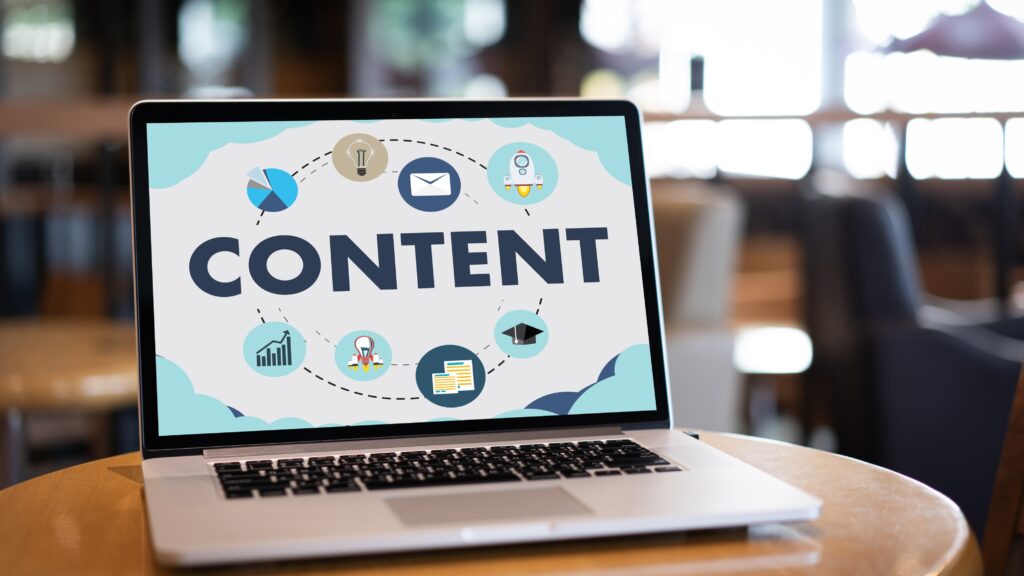
Data Anonymization and Privacy
Generative AI is used for data anonymization, a crucial aspect of data privacy. By generating synthetic data that retains the statistical characteristics of the original dataset but doesn’t contain personally identifiable information, organizations can conduct data analysis without violating privacy regulations.
Data Augmentation for Machine Learning
In machine learning, having a diverse and extensive dataset is essential. Generative AI can be used to augment existing datasets by generating variations of the available data. This approach can lead to improved model performance and generalization.
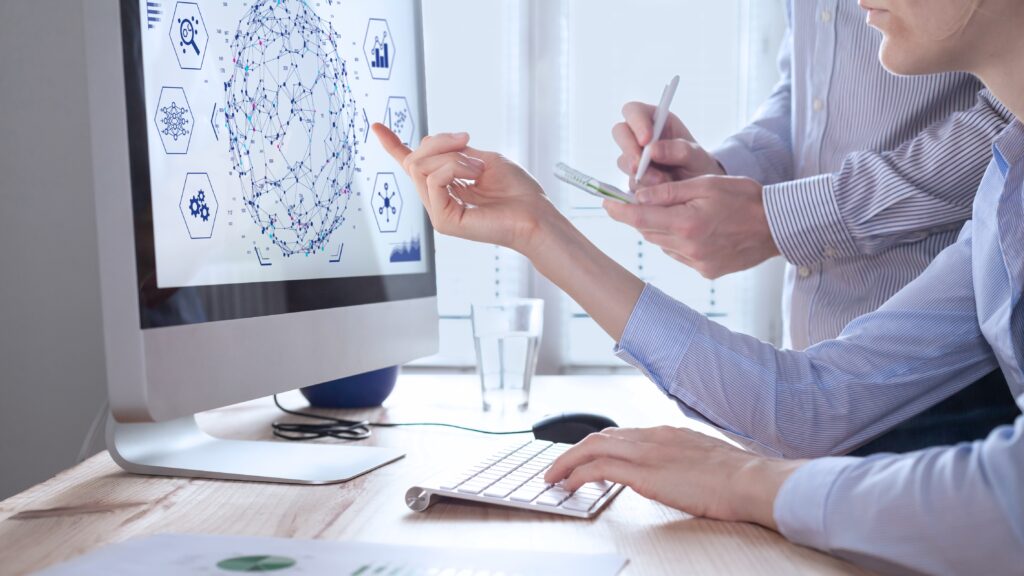
Predictive Modeling
Data engineers are responsible for feeding data into machine learning models. Generative AI can be used to generate predictions or forecasts based on historical data patterns, which can then be integrated into decision support systems.
Enhanced Data Analysis
Generative AI can be employed to create synthetic data points for analysis. Data engineers can use these data points to validate models, test hypotheses, and perform scenario analysis without the constraints of real-world data limitations.
Automating Repetitive Tasks
Generative AI can automate repetitive data engineering tasks, such as data integration and cleansing. This reduces the workload on data engineers, allowing them to focus on more complex and strategic activities.
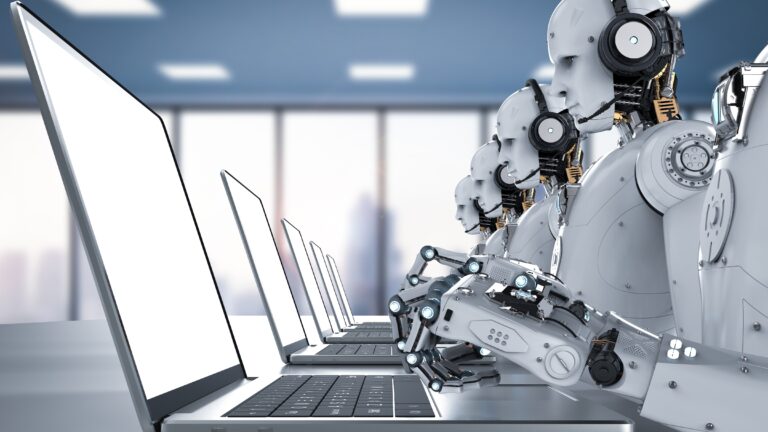
Challenges and Considerations
While the collaboration between data engineering and generative AI is promising, it’s not without challenges:
1. Quality Control
The quality of data generated by AI models must be rigorously assessed to ensure it is consistent with real data. Inaccurate or misleading data could have severe consequences, particularly in domains like healthcare or finance.
2. Data Bias
Generative AI models can inadvertently perpetuate biases present in the training data. Data engineers and data scientists must be vigilant in detecting and mitigating such biases, especially when using AI-generated data for decision-making.
3. Security Concerns
The use of generative AI to generate synthetic data must adhere to strict security and privacy standards. Failing to protect generated data can lead to data breaches and legal repercussions.
4. Ethical Considerations
Ethical considerations, particularly in content generation, are essential. The use of generative AI to produce text or other content that may deceive or mislead consumers raises ethical questions.
5. Human-Machine Collaboration
Successful integration of generative AI into data engineering requires a well-defined collaboration between data engineers, machine learning engineers, and domain experts. Human oversight is necessary to ensure generated data aligns with the desired objectives and complies with standards.
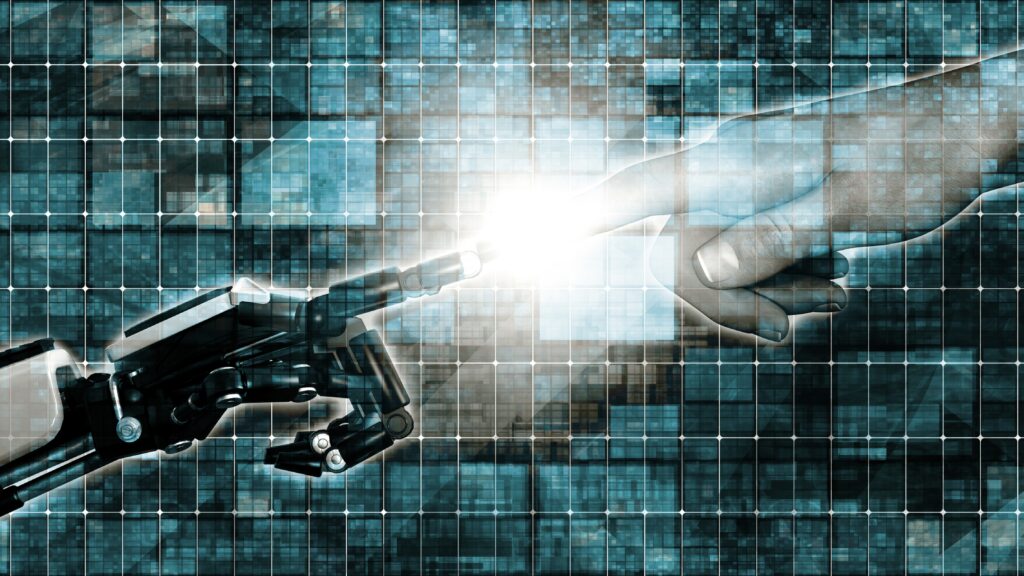
The Future of Data Engineering and Generative AI
The future of data engineering and generative AI is a landscape of tremendous potential. Some aspects to watch for in this evolving field include:
1. Increased Efficiency
As generative AI becomes more integrated with data engineering processes, efficiency gains will be realized across data preparation, data generation, and data analysis.
2. AI-Powered Tools
The proliferation of AI-powered tools for data engineering will enhance the capabilities of data professionals. Expect to see a range of applications for data quality improvement, data augmentation, and data anonymization.
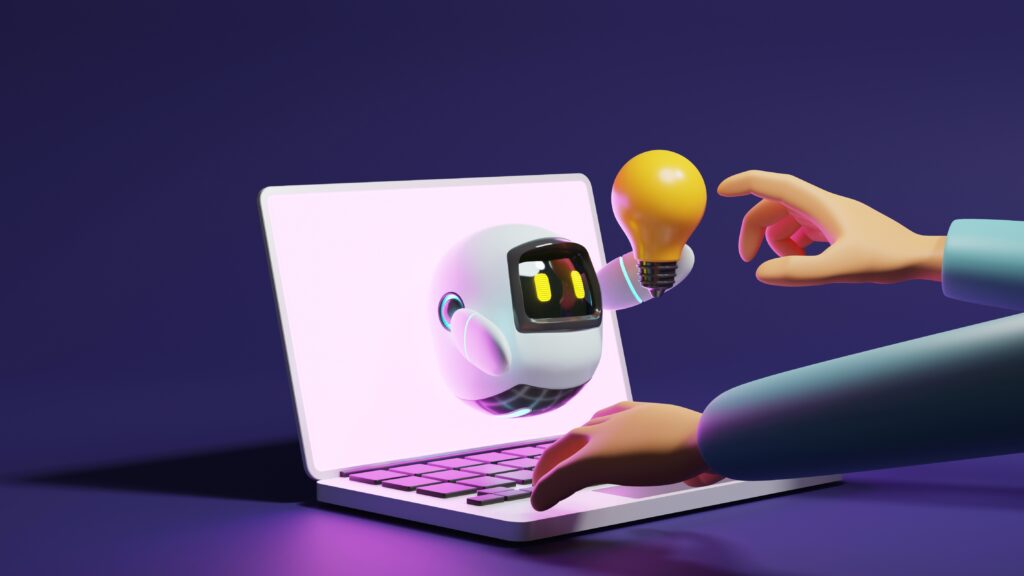
3. Wider Adoption
Generative AI will become more accessible and widely adopted in data engineering tasks. Organizations of all sizes will incorporate these technologies into their workflows, benefiting from improved data quality, enhanced content generation, and better decision-making support.
4. Greater Accuracy
Improvements in AI models’ accuracy, bias mitigation, and ethical considerations will lead to more reliable and trustworthy data generation and content creation.
5. Creative Collaboration
The collaboration between data engineers and generative AI will extend to creative content generation. This will have a profound impact on industries like marketing, advertising, design, and entertainment.
Conclusion
The intersection of data engineering and generative AI is a testament to the transformative power of technology. It offers the potential to revolutionize data preparation, content generation, and data analysis across various domains. While there are challenges to address, the benefits are clear: increased efficiency, enhanced content creation, and a broader range of data-driven possibilities. As data professionals continue to harness the capabilities of generative AI, we can anticipate a future where data engineering becomes more efficient, creative, and impactful than ever before.
The journey ahead is one of exploration, collaboration, and innovation. Data engineers, data scientists, and AI developers will play a pivotal role in shaping this exciting convergence of data engineering and generative AI. Together, they will unlock new dimensions of data-driven insights and creative content that will define the technological landscape of tomorrow.
IBU Consulting, at the forefront of data innovation, stands ready to guide organizations on this transformative journey, ensuring they harness the full potential of data engineering and generative AI while maintaining the highest standards of quality and ethics.